Sheng Zhong - SUNY at Buffalo
Students: Spring 2025, unless noted otherwise, sessions will be virtual on Zoom.
PrivacyEnhancing k-Anonymization of Customer Data
Apr 27, 2005
Download:
Abstract
In order to protect individuals' privacy, the technique ofk-anonymization has been proposed to de-associate sensitive
attributes from the corresponding identifiers. In this work,
we provide privacy-enhancing methods for creating k-anonymous
tables in a distributed scenario. Specifically, we consider a
setting in which there is a set of customers, each of whom
has a row of a table, and a miner, who wants to mine the en-
tire table. Our objective is to design protocols that allow the
miner to obtain a k-anonymous table representing the
customer data, in such a way that does not reveal any
extra information that can be used to link sensitive
attributes to corresponding identifiers, and without
requiring a central authority who has access to all the
original data. We give two different formulations of
this problem, with provably private solutions. Our solutions
enhance the privacy of k-anonymization in the distributed
scenario by maintaining end-to-end privacy from the original
customer data to the final k-anonymous results.
About the Speaker
Sheng Zhong received his Ph.D in computer science from
Yale University in the year of 2004. He holds an assistant
professor position at SUNY Buffalo and is currently on
leave for postdoctoral research at the Center for Discrete
Mathematics and Theoretical Computer Science (DIMACS).
His research interests, on the practical side, are
security and incentives in data mining, databases, and
wireless networks. On the theoretical side, he is interested
in cryptography and game theory.
Yale University in the year of 2004. He holds an assistant
professor position at SUNY Buffalo and is currently on
leave for postdoctoral research at the Center for Discrete
Mathematics and Theoretical Computer Science (DIMACS).
His research interests, on the practical side, are
security and incentives in data mining, databases, and
wireless networks. On the theoretical side, he is interested
in cryptography and game theory.
Ways to Watch
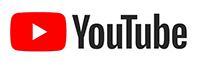